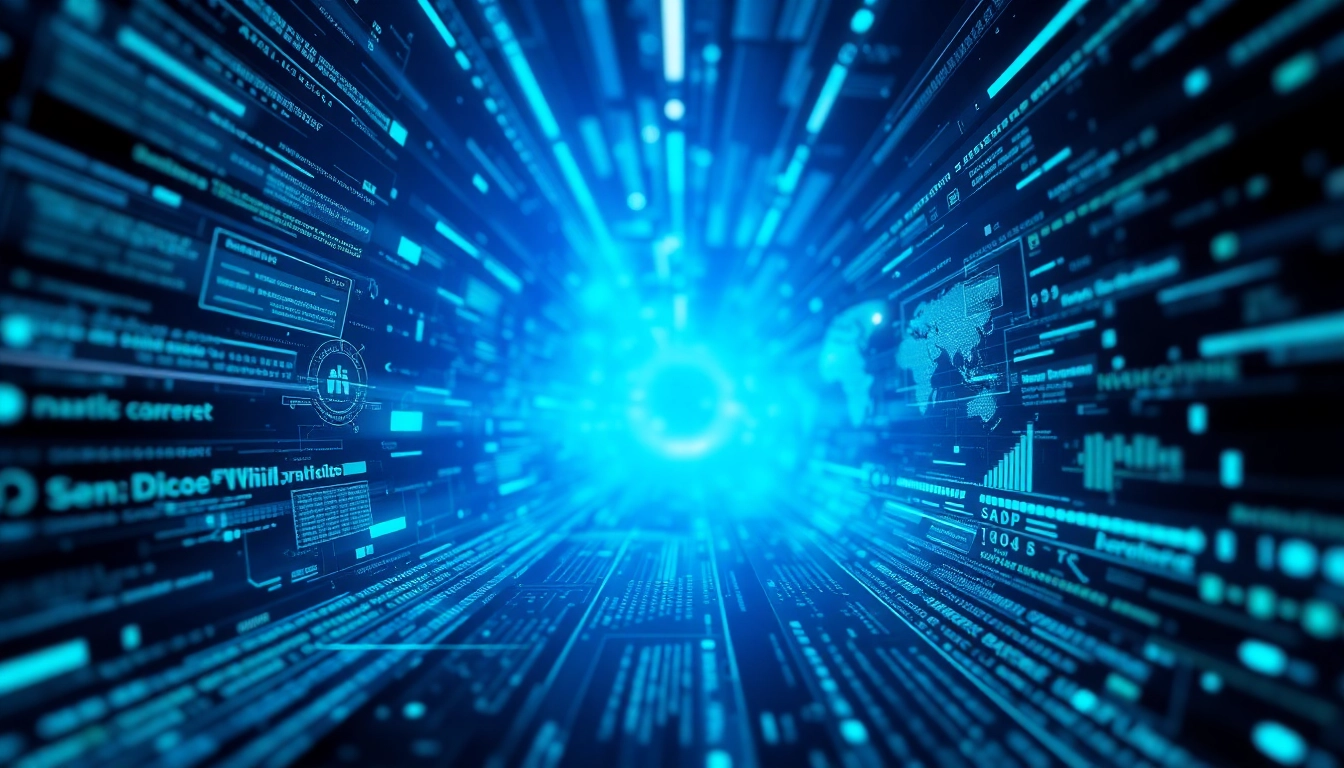
Understanding AI Search: An Overview
Artificial Intelligence (AI) is revolutionizing the way we access and interact with information. AI search technologies are at the forefront of this transformation, enhancing traditional search engines with capabilities that allow for deeper understanding and more accurate retrieval of data. Understanding AI search is essential for businesses and individuals looking to optimize their information retrieval processes. The pivotal aspect of AI search lies in its ability to interpret complex queries and deliver human-like results. By implementing AI search, organizations can significantly improve user experience and satisfaction.
What is AI Search?
AI search refers to search technologies that utilize artificial intelligence techniques, such as machine learning, natural language processing, and deep learning, to enhance the search process. Unlike traditional search engines that rely heavily on keyword matching, AI search systems analyze the context and intent behind user queries. This results in more relevant and personalized search results, which are particularly beneficial in environments rich with data, such as enterprise systems, e-commerce platforms, and content management systems.
The Evolution of Search Technology
The journey of search technology has been remarkable. Early search engines like AltaVista and Yahoo! relied on basic keyword indexing. The introduction of Google brought about a paradigm shift with its PageRank algorithm, emphasizing back-links and content relevance. As the internet evolved, so did user expectations. The rise of AI search can be traced to demands for faster, more relevant results, as well as the explosion of unstructured data across the web. Companies like Microsoft and Google are now integrating AI to provide users with a seamless search experience, catering to the need for efficiency and accuracy.
Key Benefits of AI-Driven Searching
- Improved Accuracy: AI search leverages complex algorithms to understand nuances in language, resulting in higher accuracy in search results.
- Contextual Understanding: By analyzing user behavior and query context, AI search can deliver more relevant results tailored to individual needs.
- Enhanced User Experience: Speed and relevance in search results improve the overall user experience, leading to increased satisfaction and engagement.
- Automation of Information Retrieval: AI search systems can automate browsing and information retrieval, saving time and resources for users.
How AI Search Works
Back-end Technologies Behind AI Search
AI search systems are underpinned by a range of technologies that work together to deliver optimized search capabilities. These include:
- Machine Learning: Algorithms enable search engines to learn from user interactions, improving the search over time.
- Natural Language Processing (NLP): NLP facilitates the understanding of human language by breaking down user queries, identifying intent, and extracting keywords.
- Data Mining: AI search uses data mining techniques to derive patterns from large datasets, aiding in prediction and recommendation systems.
The Role of Natural Language Processing (NLP)
NLP is crucial in AI search, as it allows machines to process human language input. This technology helps differentiate between intent and context, enabling the system to interpret queries more effectively. For example, if a user asks, “What’s the weather like in New York tomorrow?” NLP allows the search engine to recognize this is a request for forecast data specific to a location and date, rather than taking the words literally. Recent advancements in NLP, exemplified by models like OpenAI’s ChatGPT, have significantly improved the nuances of understanding queries.
Integrating Machine Learning in Search Systems
Machine Learning (ML) is the backbone of AI search technologies. By analyzing vast amounts of data, ML algorithms can identify patterns and trends that inform search results. For instance, based on user behavior, an AI search engine can learn to prioritize certain types of content or understand when users prefer short answers versus detailed explanations. The iterative nature of ML means that these systems continually refine their output as they process more queries, leading to progressively improved user experiences.
Comparative Analysis of Leading AI Search Platforms
Review of the Top AI Search Engines
As AI search technology matures, various platforms have emerged, each with unique strengths:
- Google Search: Google leverages its immense data and advanced algorithms to provide rich search results, incorporating features such as featured snippets, knowledge graphs, and more.
- Bing AI: Microsoft’s Bing is increasingly focusing on integrating AI capabilities, improving image searches and contextual responses.
- Amazon Kendra: Designed for enterprises, Kendra brings together machine learning and natural language understanding, enabling organizations to extract insights from various data sources.
- ChatGPT Search: This innovative search tool allows users to pose questions in natural language, retrieving instant answers and linking to relevant sources.
Performance Metrics and User Experience
When evaluating AI search platforms, various performance metrics should be considered:
- Response Time: The speed at which search results are delivered.
- Relevance of Results: Measured by user engagement and satisfaction with search outcomes.
- Adaptability: The ability of the search engine to learn from user behavior and improve over time.
Understanding these metrics not only helps in selecting a suitable AI search platform but also guides improvements in the User Experience (UX) design of the application.
Case Studies: Success Stories from Industry Leaders
Numerous organizations have successfully implemented AI search technology to boost operational efficiency and customer interactions. For example:
- Slack: Enhanced its internal search capabilities to allow employees to find relevant discussions and documents quickly, leading to a more efficient workflow.
- eBay: Utilized AI search technologies to improve product discoverability, resulting in increased conversion rates by personalizing search experiences.
- Netflix: Leveraged AI search to analyze user behavior and preferences, personalizing recommendations, which has been a critical factor in customer retention.
Implementing AI Search for Your Business
Identifying Search Needs and User Intent
The first step in implementing AI search technology is to assess your organization’s specific needs. Understanding user intent is crucial; this involves conducting surveys and gathering data to see what users are searching for and the context around those queries. By leveraging tools like heatmaps and user journey analytics, businesses can gain insights into how users interact with existing search functionalities and identify areas for improvement.
Steps to Incorporate AI Search Solutions
- Assessment: Evaluate existing search infrastructure and determine gaps.
- Vendor Selection: Research and select AI search solutions that align with organizational goals.
- Customization: Tailor the AI search system to suit your content types and user personas.
- Testing: Run pilot tests to identify potential user interface enhancements.
- Deployment: Roll out the solution across your organization with training for end-users.
Measuring Impact and Continuous Improvement
After implementing AI search, it is critical to measure its impact. This involves tracking key performance indicators (KPIs) such as user engagement rates, search completion times, and user feedback. Continuous improvement should be a recurring process, with regular updates to the algorithms based on changing user behavior and data trends. Feedback loops are fundamental to ensuring the search system adapts and evolves, providing optimal search performance.
The Future of AI Search Technology
Trends Shaping the Next Generation of Search
The landscape of AI search technology is rapidly changing. Key trends include:
- Voice Search: With the increasing use of voice-activated devices, optimizing for voice search is becoming essential.
- Multimodal Search: The integration of text, image, and voice search capabilities is becoming more seamless, allowing users to search using various modalities.
- Personalization: Continued advancements in AI will drive hyper-personalized search experiences based on user habits and preferences.
Potential Challenges and Solutions
As AI search technology develops, several challenges may arise, including:
- Data Privacy: Ensuring compliance with regulatory requirements while leveraging user data for search improvement.
- Bias in AI Models: Continually monitoring and refining algorithms to mitigate bias in search results.
- Integration Complexity: Finding ways to integrate new search technologies with legacy systems can pose challenges; thorough planning and incremental deployment strategies can mitigate these risks.
Preparing for a Rapidly Evolving Landscape
To stay competitive in a fast-paced digital world, businesses must remain agile and open to the rapid evolution of AI search technologies. Regular training and upskilling of teams involved in search technology, alongside staying informed about industry advancements, are key strategies that organizations should adopt. Moreover, fostering a culture of innovation within the teams can help anticipate future search trends and explore new methodologies effectively.